Tech Press Review - Sept 6th 2023
---
In a thought-provoking examination of the intersection between AI and intellectual property, the author explores the complexities that arise when AI is used to reproduce or mimic styles in music and art among others. Current technology could soon allow a smartphone application to imitate a specific artist’s voice perfectly. Although this raises concerns regarding intellectual property rights and who should be paid, it's clear that as technology evolves, so too must our understanding of related legalities and norms.
Further complexity ensues when different perceptions of cultural appropriation are brought into the spot. For instance, in the art world, mimicking another artist’s style does not necessarily equate to theft. Opinions differ greatly, underscoring the need for a consensus that will not be simple to reach in the world of AI.
The article also discusses the issues evolving around news summaries created by AI. Although legalities around this direct reproduction of content are hazier, it's highlighted that an algorithmic summary doesn’t equate to theft. However, AI technology raises concerns about scalability and the possibility of such technology superseding the need for original content.
With AI's ability to infer patterns from vast amounts of data, there's an emerging debate surrounding the ownership of this data. Despite individual contributions being minute, they form part of a larger, essential dataset. AI’s dependency on such collective input raises questions: should all contributors be compensated, or should the laws around fair use be adapted?
Lastly, the author emphasizes that AI is merely a tool in the creation process, and while it might aid us in producing art or music, human skill and creativity remain paramount in creating quality content. While today’s AI may still be in its early stages, the promise of tomorrow’s advancements is tantalisingly unknown.
Source => https://www.ben-evans.com/benedictevans/2023/8/27/generative-ai-ad-intellectual-property
---
Meta AI has developed DINOv2, a new method for training high-performance computer vision models, which is capable of generating high-quality video segmentation. This innovation demonstrates significant progress from the original DINO method as it offers a robust understanding of object parts and both semantic and low-level understanding of images.
Unlike most recent reconstruction-based self-supervised learning methods, DINOv2 does not require fine-tuning and can deliver high-performance attributes directly used as inputs for simple linear classifiers. The versatility of DINOv2 allows it to be applied in creating multi-purpose backbones for different computer vision tasks, including classification, segmentation, and image retrieval.
The training of DINOv2 is implemented using self-supervised learning, a method that does not require vast amounts of labeled data. This implies that DINOv2 can be trained using any collection of images without any associated metadata required. Additionally, DINOv2 can learn features that the current standard approach fails to interpret, such as depth estimation.
Considering its remarkable out-of-domain performance attributed to the combination of self-supervised feature learning and the use of lightweight task-specific modules, DINOv2 models are expected to be beneficial in various applications. Meta has collaborated with the World Resources Institute in using AI to map forests across large areas. The self-supervised model was trained on data from forests in North America, and proven to generalize well and produced accurate maps in other locations worldwide.
Finally, due to the stable and scalable training offered by DINOv2, the potential for further advancements in applicative domains is vast. Such an application already in progress is the forest-mapping collaboration with the World Resources Institute. Transitioning to DINOv2 involved overcoming several challenges such as creating extensive and well-curated training datasets, enhancing the training algorithm, and coming up with a functional distillation pipeline.
Source => https://ai.meta.com/blog/dino-v2-computer-vision-self-supervised-learning/
---
In a breakthrough study by computer vision researchers Leonid Keselman and Martial Hebert, fast and reliable shape reconstruction, critical in several computer vision applications, has seen significant advancements. They've built upon current methods of differentiable rendering with 3D Gaussians - a shape representation alternative.
The pair have developed extensions to the previous work in this area, adding aspects such as integrating differentiable optical flow, exporting watertight meshes, and rendering per-ray normals. Noteworthy are the improvements to the quickness and robustness of these reconstructions, which could now be effortlessly performed on either a GPU or CPU.
Further elaboration on their work includes examples of optimizing shape reconstruction from a CO3D video sequence. They completed this using forty 3D Gaussians, a stark display of the practical usefulness of these techniques. Another contribution was the application of differentiable optical flow in aiding the reconstruction to yield more precise shapes.
Their work also brings interoperable qualities, blending Fuzzy Metaballs and 3D Gaussian Splatting both using 3D Gaussians as the base for shape representations. Demonstrating this, they performed a 3DGS reconstruction of a ficus tree and exported it through their mesh exporting pipeline. This type of work offers notable potential for the future applications of the technology, as it can be exported and rendered externally, using software like Blender.
In conclusion, the described advancements in differentiable rendering with 3D Gaussians are set to drive the development in various computer vision applications while granting better efficiency and accuracy in shape reconstructions.
Source => https://leonidk.com/fmb-plus/
---
In a recent venture, Google Lab Sessions collaborates with Grammy award-winning rapper and MIT visiting scholar, Lupe Fiasco, on an experimental AI project named TextFX. The project is designed to assist in crafting ingenious linguistic constructs and pursuing creative text possibilities, highlighting the potential of AI in enhancing human creativity.
Through the study of Lupe’s creative approach, Google developers aimed to utilize AI in optimizing his unique technique of creating phonetically identical phrases with distinct meanings. They leveraged Large Language Models (LLMs), specifically designed to perform language-related tasks, and tuned them to fit Lupe’s lyric-writing workflow.
Models like Google’s Bard function as conversational agents, while others like the PaLM API’s Text Bison extend or completely fulfill a given input text. The experiment benefited from the latter's ability for few-shot learning, recognizing and replicating patterns from a small set of examples. Lupe provided examples of his inventive phrase technique, enabling them to craft suitable prompts to guide the LLMs.
Through iterative experimentation, they finally developed a successful few-shot prompt to task the model with generating same-sounding phrases. Joining forces with Lupe, they identified additional creative ventures that can be achieved with the same prompting strategy, resulting in ten unique prompts for exploring creative possibilities.
Once the prompts were finalized, they developed an app, TextFX, to house them. TextFX demonstrates the potential for AI-powered creativity, targeting creative writing, and hinting at its possible extension into other creative spheres.
Google encourages others to experiment on their platform with TextFX, while providing the open-sourced code for further exploration. The company's AI tools are bringing ideas to life, reimagining collaborations in craft, and offering a glimpse of the future in AI augmented creativity.
Source => https://developers.googleblog.com/2023/08/how-its-made-lupe-fiasco-text-fx.html
---
Over the recent years, the impressive development in large language models (LLMs) like GPT-3 and PaLM has fueled discussions on their ability to reason symbolically. The crux of the issue is whether LLMs can manipulate symbols based on logical rules. Despite their ability to deliver in diverse circumstances, LLMs falter when it comes to executing simple arithmetic operations, especially with large numbers. Even the latest model, GPT-4, continues struggling with arithmetic and calculation mistakes.
In the paper “Teaching Algorithmic Reasoning via In-Context Learning”, an approach that uses in-context learning to augment algorithmic reasoning in LLMs is introduced. In-context learning refers to a model’s capacity to perform a task after witnessing a few task examples within the model's context. Task information is passed over to the model through a prompt, eliminating the need for weight updates. With a novel algorithmic prompting technique and judiciously chosen prompting strategies, the model can solve more complex arithmetic problems while ensuring generalization.
To teach a model an algorithm as a skill, the approach makes use of algorithmic prompting that not only outputs the necessary steps for an algorithmic solution but also provides detailed explanations of each step to avoid misunderstandings. For instance, in teaching models to perform a two-number addition, the model is facilitated through each step of the computation with explicit equations.
The approach's efficacy is further demonstrated in its application to grade school math word problems (GSM8k). Here, differently-prompted models interact to perform complex tasks. For instance, one model might specialize in informal mathematical reasoning while another may excel in addition calculations. They work in tandem, the former takes the lead, and whenever an arithmetic operation is required, it calls on the latter to perform it.
Overall, this strategy showcases a promising path forward in LLM development by leveraging in-context learning and novel algorithmic prompting, potentially translating advanced reasoning performance into longer contexts with detailed explanations.
Source => https://ai.googleblog.com/2023/08/teaching-language-models-to-reason.html?m=1
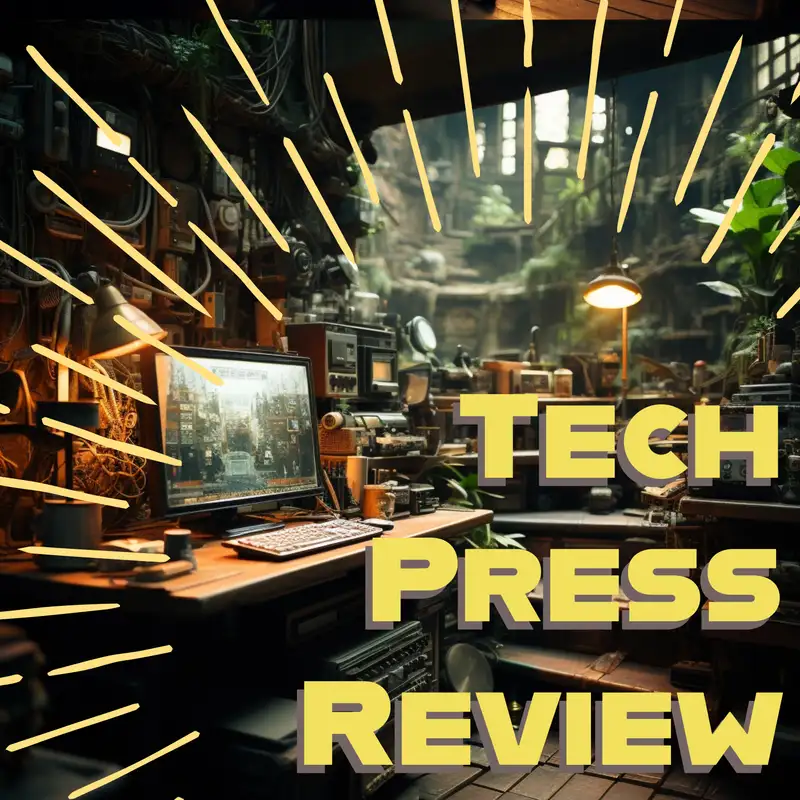